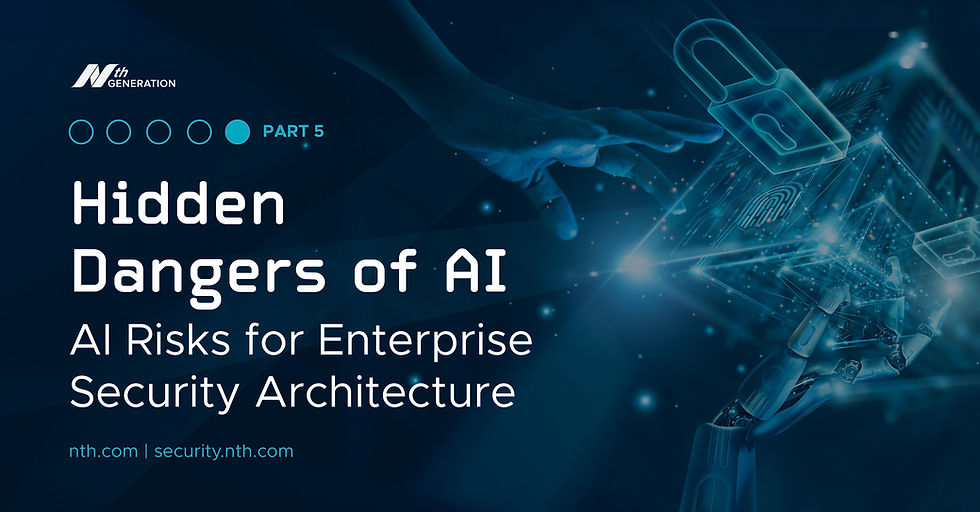
In this last of a five-part series, we can see how the evolving landscape of enterprise security can integrate artificial intelligence (AI) into security architecture and how it presents both significant opportunities and challenges.
This series of articles outlines a comprehensive approach to safeguarding AI systems through robust technical measures and governance frameworks. It emphasizes the importance of multi-layered security strategies including regular audits, data encryption, and stringent access controls to protect against threats like industrial espionage and ransomware. These articles further advocate user-visible policies, transparent AI models, and open responsibility frameworks to foster accountability and ethical practices. It highlights the need for resilient system designs and a controlled, phased approach to AI adoption to mitigate risks. By balancing technological advancements with responsible governance, organizations can effectively secure their AI infrastructure and ensure ethical, transparent, and safe operations.
AI in Enterprise Security Architecture
Implement Robust Technical Security Measures
Multi-Layered Security: Multiple security layers are crucial for protecting AI systems and infrastructure. This "defense in depth" approach helps prevent data loss, including intellectual property and personal information. With rising concerns about industrial espionage and ransomware, employing layered defense mechanisms like firewalls, intrusion detection systems, and endpoint protection can strengthen security. Regular updates and integration with AI-driven tools enhance effectiveness by spotting and addressing threats in real-time.
Regular Audits and Updates: Regular security audits are vital for finding vulnerabilities in systems and networks. Audits should cover all IT infrastructure aspects, with prompt updates to address any weaknesses. Organizations should schedule routine audits with both internal and external experts, using AI tools for continuous monitoring and automated scanning. This proactive approach helps prevent risks before they can be exploited. Updates based on vendor updates should follow a staged scenario.
Enhance Transparency and Accountability
Transparent AI Models: Developing AI models that are explainable and transparent is crucial for fostering trust and accountability. Such models allow stakeholders to understand decision-making processes, making it easier to identify biases and ensure fair outcomes, particularly in critical areas like finance, healthcare, and law enforcement. Techniques like explainable AI (XAI) provide clear and interpretable outputs, enabling stakeholders to verify the accuracy and fairness of AI systems. By adopting transparent AI models, organizations can enhance trust among users and regulators, ensuring ethical and responsible AI usage.
Clear Responsibility Frameworks: Establishing clear frameworks that outline the responsibilities of AI systems and human operators is essential for ensuring accountability and adherence to ethical guidelines throughout the AI lifecycle. Moreover, enacting controls over the execution of the responsibilities be they human policies or AI guardrails and constraints provides verifiable safeguards for responsible behavior.
Ethical Guidelines: Organizations should create policies that specify the roles and responsibilities of AI developers, users, and oversight bodies, addressing issues such as data privacy, security, and ethical considerations. By doing so, they can minimize the risk of misuse or harm and ensure that AI systems are developed and used responsibly.
Strengthen Data Protection
Data Encryption: Encrypting sensitive data both in transit and at rest is crucial for protecting it from unauthorized access. As AI systems increasingly handle large volumes of sensitive data, ensuring its encryption is vital for maintaining privacy and security. This includes encrypting data during transmission between systems and when stored on servers. AI platforms should support encryption by default, simplifying the protection of data. By prioritizing data encryption, organizations can safeguard sensitive information and comply with data protection regulations.
Access Controls: Strict access controls are essential for limiting who can view or modify sensitive information. These controls ensure that only authorized personnel have access to critical data, reducing the risk of breaches and unauthorized changes. Role-based access control (RBAC) and multi-factor authentication (MFA) are effective methods for enhancing security. AI-driven access control systems can dynamically adjust permissions based on user behavior and context, providing an additional layer of security. Robust access controls protect sensitive data and maintain its integrity.
Monitor and Regulate AI Usage
Data Usage Policies: Developing and enforcing policies on AI system use is essential to prevent the inadvertent upload of sensitive information. These policies should clearly define acceptable AI uses and specify the types of data that can be processed by AI systems. Ensuring compliance with these policies helps protect sensitive information and maintain ethical AI practices. Organizations should train employees on data usage policies and the importance of protecting sensitive information.
Transparency and Accountability: Transparency and accountability in AI systems are crucial for maintaining trust and compliance. Organizations should implement mechanisms for tracking and auditing AI activities, ensuring all actions are recorded and reviewable. This helps identify and address misuse or unethical practices.
Compliance with Regulations: Acquisition and use of security tools and platforms that ensure compliance with data protection regulations like GDPR, CCPA/CPRA, and others provide protection of sensitive data and demonstrate due care.
Ethical AI Development: Promote ethical AI development practices that prioritize privacy, security, and transparency. Remember that many of the AI platforms allow for added personal creation of AI constructs, most notably chatbots. Since employees can and may be creating their own AI instances, adherence to ethical practices must be closely followed and monitored.
Invest in Resilient Systems
Redundancy and Backup Systems: Creating redundant systems and backups ensures continuity in case of AI failures. Redundancy involves having multiple copies of critical systems and data so that if one fails, another can take over without disruption. Backup systems ensure data can be restored in case of loss or corruption. Organizations should regularly test redundancy and backup systems to ensure they function as expected. AI-driven monitoring and recovery tools can enhance system resilience by providing real-time alerts and automated recovery processes. Investing in redundancy and backup systems minimizes downtime and maintains operational continuity.
Resilient Design: Designing AI systems to be resilient against attacks and failures is crucial for maintaining their reliability and security. Resilient design incorporates fail-safes and recovery protocols to handle unexpected disruptions. This includes designing systems to detect and respond to attacks and implementing fallback operations using non-AI tools or manual processes. Organizations should conduct regular stress testing and scenario analysis to identify potential vulnerabilities and improve AI system resilience. A resilient design approach ensures AI systems remain operational and secure, even in the face of challenges.
Implement a Controlled, Phased, Risk-based Approach to AI Adoption
Laboratory Environment Testing: Starting with AI experimentation in a controlled "laboratory" environment allows organizations to simulate critical processes and sensitive data type content without risking real-world operations. This helps identify potential issues and refine AI models before deployment. Organizations should gradually expand AI usage by conducting organization-wide Business Impact Assessments (BIAs) and selecting non-critical areas for beta testing. A phased approach mitigates risks and builds confidence in AI systems.
Gradual Adoption: Gradually introducing AI usage across different organizational levels helps manage the adoption process and ensures employees are familiar with AI tools. This involves starting with low-impact areas and progressively moving to higher-impact areas, focusing on training and support. More specifically, organizations should prioritize areas with the least critical processes and sensitive data, slowly moving towards the areas with the most critical processes and sensitive data for the final phases of AI adoption, ensuring comprehensive risk assessments and mitigation strategies are in place. A controlled, phased approach effectively integrates AI into operations while minimizing risks.
General Mitigations
Governance, Risk, and Compliance
Robust governance frameworks help ensure responsible AI use. Organizations should establish clear policies and guidelines for data security, privacy, and ethical considerations with respect to sensitive information especially third-party data. Enhancing organizational monitoring through AI-driven tools helps track compliance and identify potential issues. Training employees on responsible AI usage and developing contingency plans for AI failures or misuse are crucial for maintaining a secure and ethical AI environment and ensuring continuity of operations. Prioritizing governance, risk, and compliance ensures AI systems are used responsibly and effectively.
Balanced Approach
A balanced approach to AI adoption considers broader societal impacts and ensures AI benefits both organizations and the wider community. Policy interventions by governments can provide frameworks for ethical AI use but organizations must take corporate responsibility for their AI deployments.
Raising public awareness about AI's potential risks and benefits helps society adapt to new technologies and ensures AI enhances human capabilities and quality of life. A balanced approach harnesses AI benefits while mitigating its potential risks.
___________
This article series provides a detailed framework for enhancing enterprise security in the context of AI integration. It advocates for a multi-layered security approach, including regular audits, data encryption, and stringent access controls to protect against various threats. Transparency and accountability in AI models are emphasized to ensure ethical use and fair outcomes. It also underscores the importance of resilient system designs and a phased adoption strategy to manage risks effectively. By combining robust technical measures with responsible governance and ethical guidelines, organizations can secure their AI systems while maintaining trust and compliance.
コメント